报告题目:Information Geometry: Geometrization of Statistical Inference
报告人:Jun Zhang (University of Michigan-Ann Arbor)
主持人:冯惠涛教授(南开大学陈省身数学研究所 副所长)
时 间:2019年5月29日星期三 下午4:00---5:00
地 点:理学院 学术报告厅 (至善楼 200)
组织单位:研究生院
承办单位:数学科学研究中心 理学院
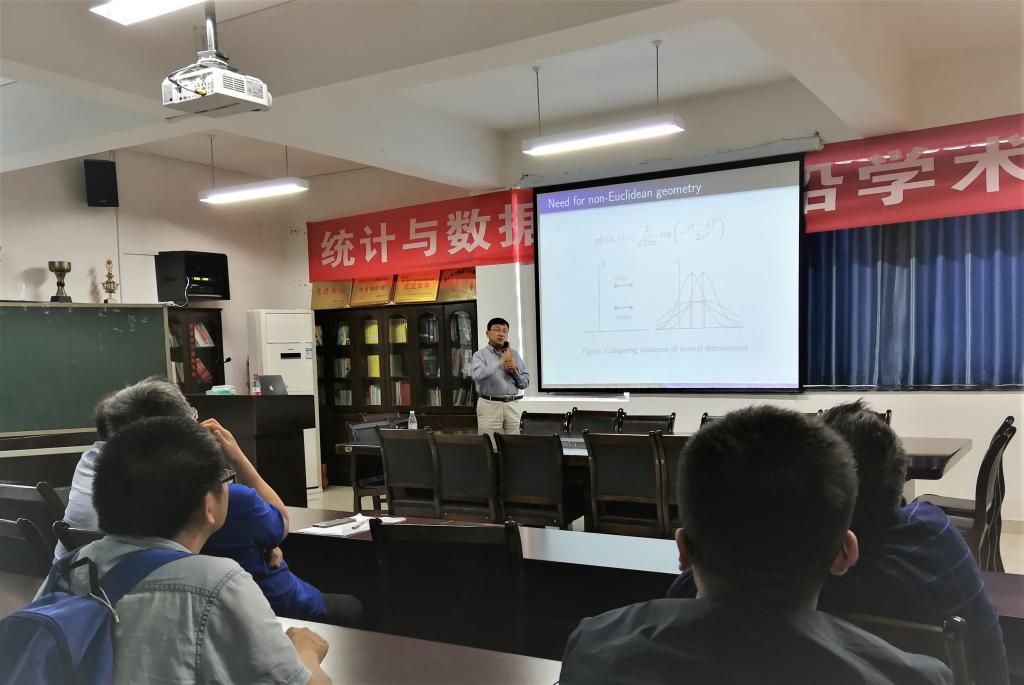
学术报告内容简介:
Information Geometry is the differential geometric study of the manifold of probability models, and promises to be a unifying geometric framework for investigating statistical inference, information theory, machine learning, etc. Instead of using metric for measuring distances on such manifolds, these applications often use “divergence functions” for measuring proximity of two points (that do not impose symmetry and triangular inequality), for instance Kullback-Leibler divergence, Bregman divergence, f-divergence, etc. Divergence functions are tied to generalized entropy (for instance, Tsallis entropy, Renyi entropy, phi-entropy) and cross-entropy functions widely used in machine learning and information sciences. It turns out that divergence functions enjoy pleasant geometric properties – they induce what is called “statistical structure” on a manifold M: a Riemannian metric g together with a pair of torsion-free affine connections D, D*, such that D and D* are both Codazzi coupled to g while being conjugate to each other. Divergence functions also induce a natural symplectic structure on the product manifold MxM for which M with statistical structure is a Lagrange submanifold. We recently characterize holomorphicity of D, D* in the (para-)Hermitian setting, and show that statistical structures (with torsion-free D, D*) can be enhanced to Kahler or para-Kahler manifolds. The surprisingly rich geometric structures and properties of a statistical manifold open up the intriguing possibility of geometrizing statistical inference, information, and machine learning in string-theoretic languages.
报告人简介:
张俊,美国密西根大学心理学系终身教授、数学系终身教授,美国心理学协会会士,心理规律协会会士。现任《信息几何学》杂志创刊联合主编。历任全美脑与行为科学协会联盟(FABBS)执行董事,数学心理学协会副主席、主席、执行委员会委员,《数学心理学》杂志副主编。1985年获得复旦大学理论物理学士,1992年获得加里福尼亚大学伯克利神经生物学博士,并获聘于密西根大学任终身教职。在学术休假年度,张俊教授在澳洲墨尔本大学、法国科学院马赛所、加拿大滑铁卢大学、日本理研脑科学研究所、英国剑桥大学、美国哈佛大学等,担任客座研究员、访问教授等席位。张俊教授主持的M3实验室(“Mind,Machine,Mathematics”)长期开展认知建模、机器学习等人机界面、累脑人工智能算法研究,持续获得美国自然科学基金会、国防部等部门的科研经费支持和支撑。